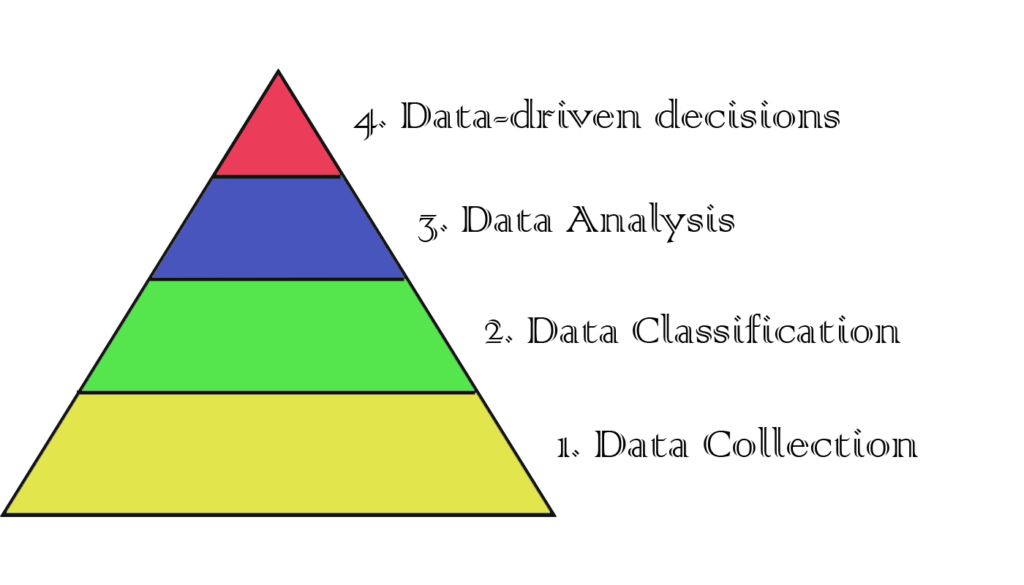
Crawling through the internet, one might get an impression that working with data is a modern way to hundred percent ensure your success on the market. The reality is not black and white, but data is truly an invaluable asset nowadays. The science around data has gone very broad and sexy, but also somehow unclear and untouchable. To make sure our customers understand how we look at their data, we’ve created a 4 phases model of data-awareness to visualize how companies should build upon their data wealth and where they need to be so that their data department or outsourced data services start to make a difference.
We decided to build a 4 phases model as we generally see exactly 4 main steps on their way to data-excellence. To be precise though, there is an extremely significant „but“. The journey through data-awareness is not a 4-stop path, rather it is very reminiscent of a Maslow’s hierarchy. If you don’t handle the lower floor well enough or your processes significantly lose quality, everything above the floor stops working for you and you start bleeding, fast and heavy.
To keep the pyramid working for you there’s a lot of hard work and investments to make, but it’s worth it. What is it though? Let’s have a look.
The pyramid consists of 4 floors:
- Data collection
- Data classification
- Data analysis
- Data-driven decisions
Let’s go through them one by one.
1. Data collection
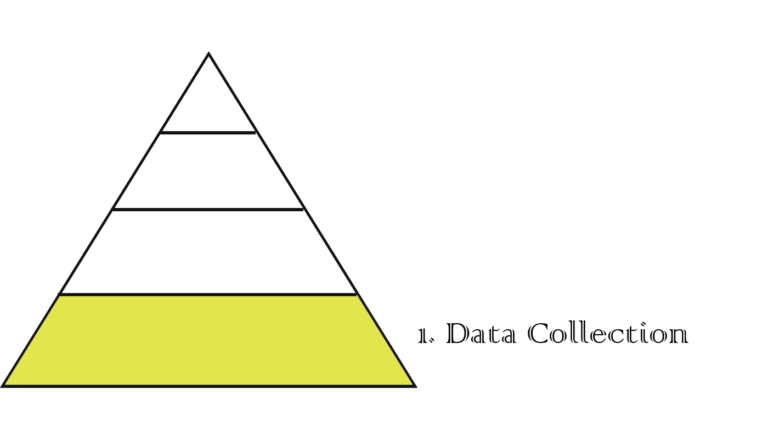
The grounding phase and an absolutely vital process in data management. That is data collection. Before starting to use data, you need to collect them. Does it seem easy? It’s not. Collecting data is easy, collecting precise data is difficult. At this point you need solid technical solutions to collect the large volumes of data reliably and have the infrastructure and storage ready for the data you collect.
Don’t forget to have a stable format of the data you collect, ideally sorted out in a special data collection directive. Your data collection should be as unified as possible not only over time, but also throughout the points of data capture.
If you manage to do the first phase well, you have a solid background for the next phases.
2. Data classification
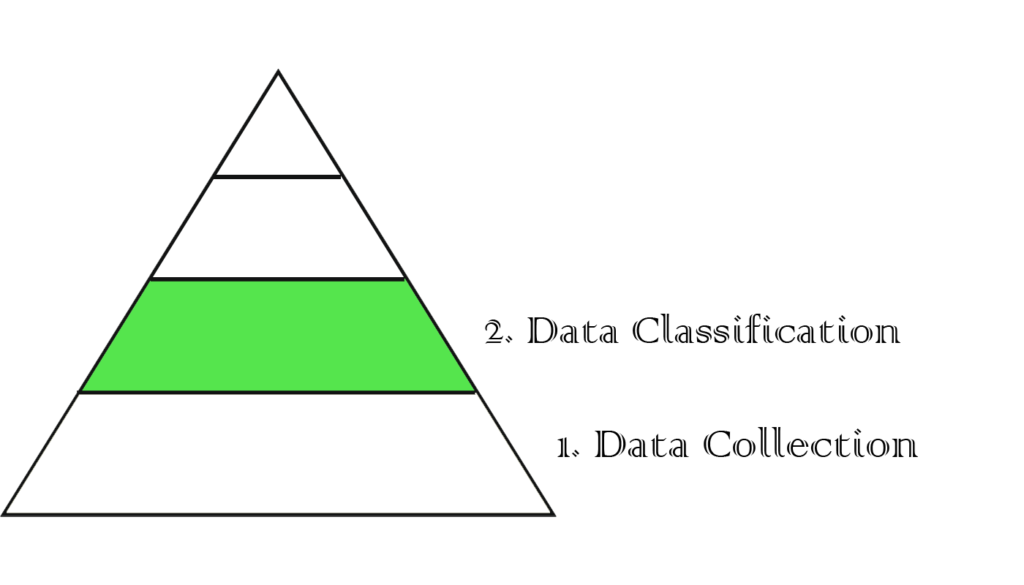
Before you start making use of the collected data, you need to maintain them. If you don’t control the quality of data and start using raw data instead of clean datasets, the information you derive through analytical methods might be biased.
Although cleaning datasets is the usual first step when approaching new data foranalysis, you should clean duplicities, incomplete or apparently faulty data before you get them to the analysts. You simply get rid of the risk of unintentionally using wrong data to make data-driven decisions, save time to your data analysts and let them search for more elaborate faults in data.
Also make sure to control whether the appropriate methods of collection are followed and the data comply with the expectations. If not, search for the reasons behind that and get back on track. If you can’t repair the collection process, change the methodology or calibrate. There’s no logical reason for spending your lifetime working with wrong or biased data.
3. Data analysis
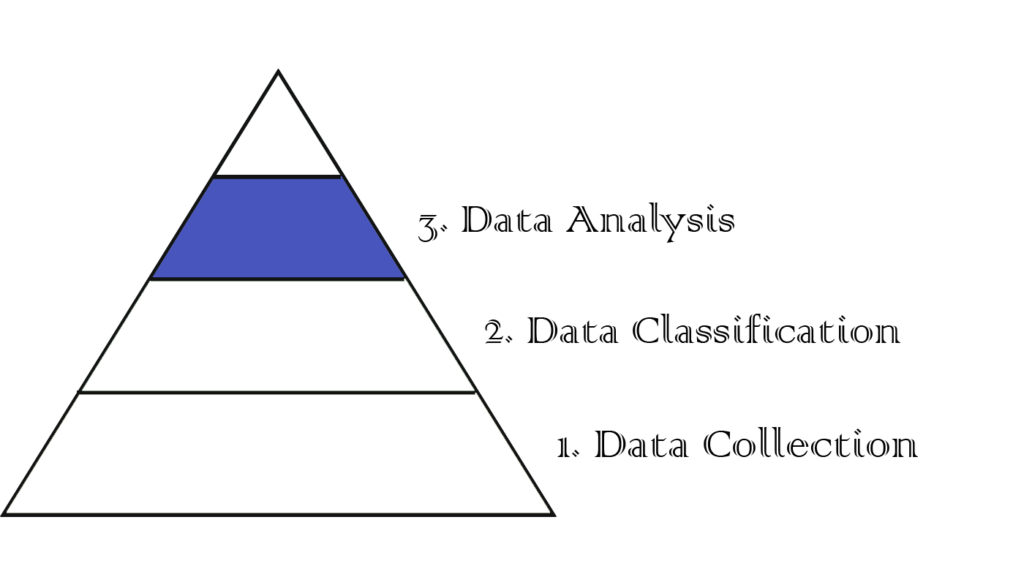
After you’ve managed to collect reliable data and maintain them, you are ready to start using them for routine analytical tasks, create dashboards and back your decisions with what you observe in your data.
Your data analysts should be able to understand not only the particular methods they use, but also have a strong background in mathematics, statistics, econometrics and other related disciplines. Many companies also expect data analysts to be business experts and be soft-skills gifted. It is always a huge benefit, if your candidate nails soft-skills, but you shouldn’t hire them just because of that. They should primarily excel at data analysis.
4. Data-driven decisions
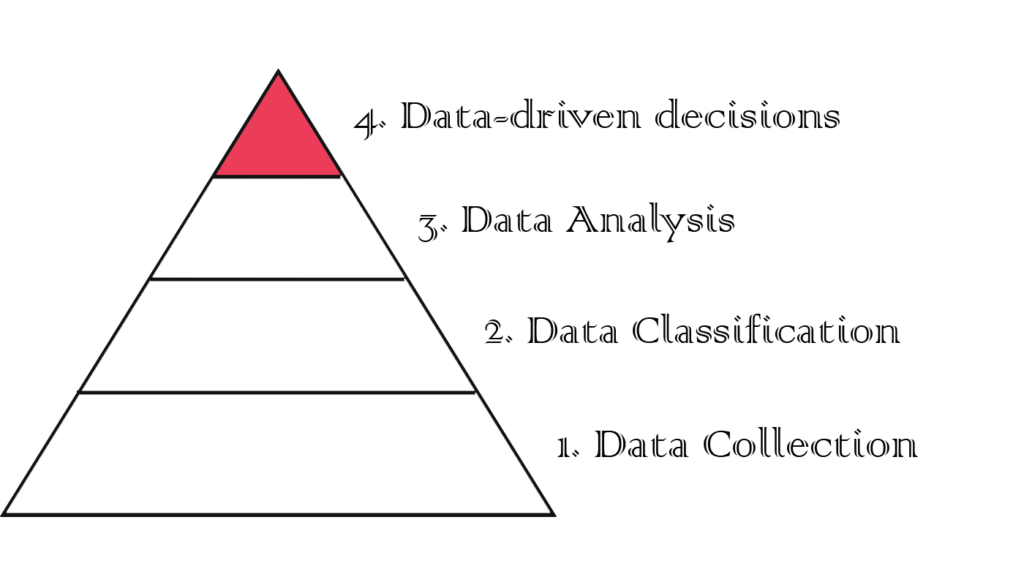
Based on the work of your data analysts, you are ready to make data-driven decisions. Dashboards, business intelligence and other statistics enable you to use data for your benefit and back your decisions with actual information, not just assumptions and beliefs.
However, sometimes you face a difficult and costly decision to be made, the problem usually is not well structured and you don’t know which data you should back your decision with. In such cases, you need a deeper analysis to find „optimum“. This is when we come in and help you with the analysis.
We are used to working with unclear tasks worth millions and although we can’t guarantee a result, in a vast majority of cases your investment saves you lots of money as you typically make a much better decision with our analysis. Sometimes your data analysts are capable enough to handle such tasks, but usually it’s not the case. Moreover, we have no stake in your company and have no motivation for any interpretation biases – we have no preffered option. Never.
Are you making such important decisions and want to do an informed data-driven decision?